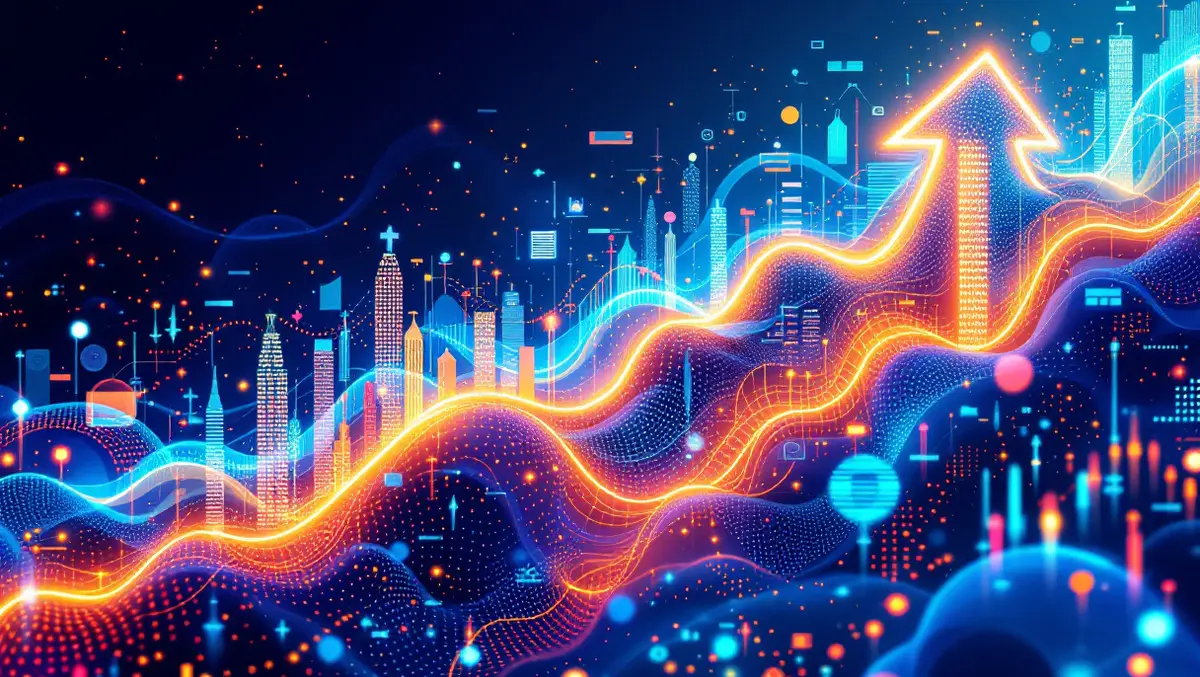
What businesses can learn from 15 years of data growth
For most of us, data leads an out-of-sight, out-of-mind existence. The problem is, people and organisations alike are, effectively, drowning in it as we create and consume digital information in near-incomprehensible volumes. Clearly, this is a situation that has been long in the making, but the snowball effect seen over the past couple of decades has created a world where, in many cases, data is generated faster than it can be managed.
To see how we arrived at this point, you only have to look back 15 years to when today's data challenges were still taking shape. In 2010, for example, self-driving cars were still at the testing stage, and the smart city concept was only just starting to receive serious attention and investment. At the same time, many businesses were measuring their data generation and storage habits in terabytes, an order of magnitude smaller than now. Perhaps most significant of all, AI was a niche set of technologies with limited real-world application outside of research labs and early-stage automation tools.
Fast forward to today, in fact, and the picture couldn't be more different. Autonomous vehicles now number in the millions, with estimates predicting over 125 million globally by 2030. There are smart cities on every continent, with China alone boasting more than 500, each producing constant data streams to integrate everything from traffic systems to public services.
Each of these trends is making a major contribution to the growth in global data volumes. In particular, the volume of unstructured data has risen exponentially since 2010 and shows no sign of slowing down. Everything from sensor readings and social media content to email archives and meeting recordings is contributing to the total, and with around 90% of business data now unstructured, the figure continues to rise with every new connected device, video file, or chatbot interaction.
But how does this play out in practical terms? When considering the volume of unstructured data generated daily, a self-driving car might produce 4-5 TB of LIDAR, camera, and AI data, while the average hospital generates over 5 TB from MRI and X-ray activities alone. At the other end of the spectrum, smart city IoT sensors are at the 50PB level, with data collected and stored from a myriad of traffic, weather, and pollution sensors.
The overall picture, therefore, is one of enormous growth. Assuming an annual compound growth rate of 30%, three pebibytes (PiB) of data stored today will grow to over 31 PiBs over the next 10 years. For context, a pebibyte is a binary measurement of computing and storage capacity and is over 12.5% larger than the more familiar petabyte. Whichever way you look at it, the numbers speak for themselves.
The need for unstructured data orchestration
Complicating matters even further is that much of today's data remains hidden in plain sight. Most organizations have only a limited view of their information assets, unable to say with certainty how much data they have, where it resides or whether it holds any real value. This is especially true of unstructured data, which is often spread across incompatible storage platforms, cloud environments and geographies. As a result, decision-makers are frequently left flying blind because they lack the insights needed to manage data proactively.
Another significant issue is the long-standing misconception that more storage is the answer to data growth. For years, the default response to rising data volumes was simply to buy more capacity. But with the pace of accumulation now being driven by machine-generated content and GenAI workloads, this strategy is increasingly unsustainable, not only inflating costs but also creating unnecessary complexity. To regain control, organizations need to shift from a device-centric to an unstructured data orchestration approach that prioritizes intelligent data management, lifecycle oversight, and policy-driven automation over reactive storage expansion.
A key part of this process involves addressing the quality and governance of data. With AI and analytics initiatives becoming more central to enterprise strategy, the risk of "garbage in, garbage out" also grows. Poorly managed, duplicated, or irrelevant data can skew insights and undermine performance. What's needed instead is a governance-led approach that ensures only high-integrity data is used for mission-critical applications. This includes everything from archiving cold files that no longer serve a purpose to ensuring that the data used to train AI models is accurate, complete and ethically sound. Done well, this creates a foundation not just for compliance, but for real competitive advantage.
These are vital considerations because, in another decade and a half, the data landscape will once again be unrecognizable from that seen today. If the last 15 years were about keeping pace with data growth, the next 15 will be about turning that growth into value.