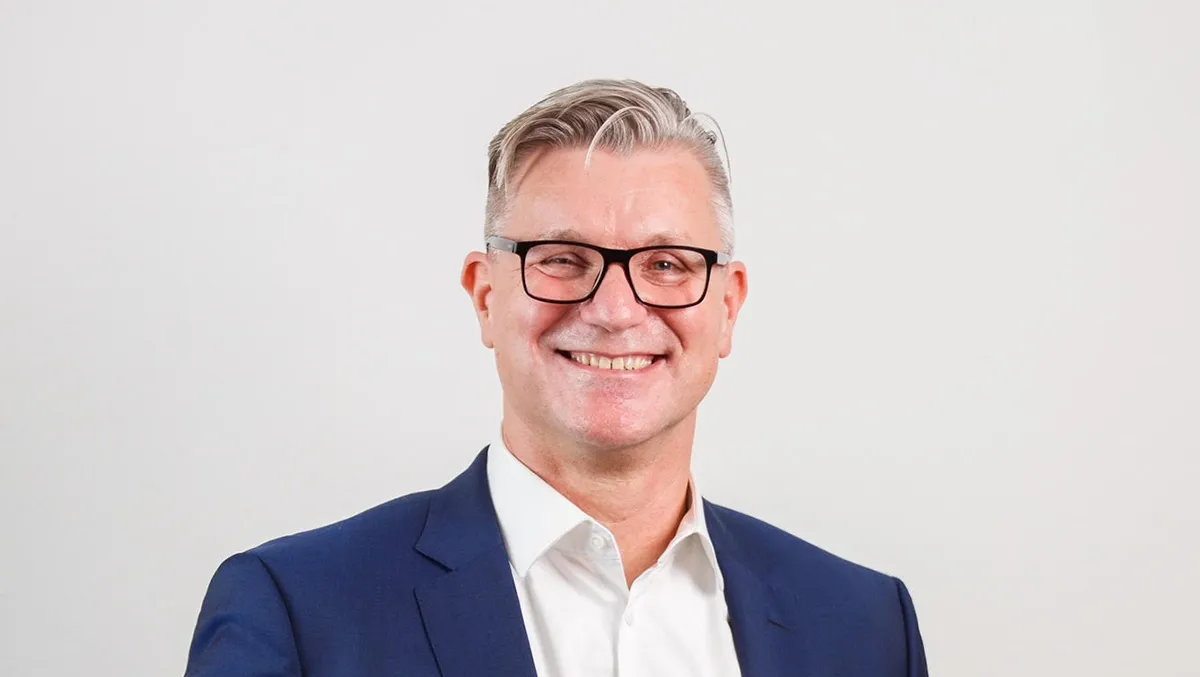
How to keep your data lake initiative from becoming a data swamp
Business leaders who leverage the many benefits of data continue to remain ahead of their competitors. Enterprises can utilise data lakes to increase agile data delivery; however, they can't reap those benefits without addressing the challenges.
The data analytics market in Australia is predicted to grow at a CAGR of 20% between 2021 to 2025. This is representative of the need for enterprises to manage vast amounts of data.
The amount of data enterprises capture daily to drive critical business decisions, improve product offerings, and serve customers better is growing faster than ever before. In 2021, the total amount of data generated in the world was upwards of 74 Zettabytes, with the projection for 2025 being more than 180 Zettabytes. To quantify this, if each Terabyte in a Zettabyte were a kilometre, it would be equivalent to 1,300 round trips to the moon and back.
But what good is all this data if companies aren't able to utilise it to guide insights in a timely manner and in accordance with their immediate goals?
Enterprises can utilise data lakes to increase data elasticity and agile data delivery; however, they cannot reap those benefits without addressing the challenges of a data lakes initiative. For example, if you try to create analytics-ready data sets from heterogeneous data manually, you'll quickly find yourself in the middle of an extremely complex (if not impossible) and time-consuming project. And when all your data is finally ready for business consumption, it's already outdated.
What is the difference between a data lake, data mart, or data warehouse?
Before getting too deep into the facts about data lakes, let's talk about the differences between a data lake, a data warehouse, and a data mart. While these types of centralised repositories all provide the ability to store data for analysis and reporting, there are some key differences when it comes to structures, data types, and functionality.
Data warehouse
A data warehouse is used for companies with a massive amount of data from specific sources, such as an ERP, core systems, or custom applications, and it is usually used for business intelligence, batch reporting, or data visualisation. Data warehouses typically have the following properties:
- They represent an abstracted picture of the business organised by subject area.
- They are highly transformed and structured.
- Data is not loaded to a data warehouse until the use for it has been defined.
- They generally follow a methodology, such as dimensional modeling and textual disambiguation.
Data mart
A data mart is basically a subset of a data warehouse where the data contained is highly accurate for specific users or data consumers. It is subject-oriented and designed to meet the needs of a specific group of users to make tactical decisions for their department. For this reason, a data mart would be of use to Australian companies with a lot of focused sales data, or perhaps a marketing department analysing customer-specific data sets from multiple sources.
Data lake
A data lake stores raw, free-flowing data, structured or unstructured, from a wide variety of sources, like social media, devices, apps, or productive databases. Their main use is for machine learning, data discovery, or predictive analysis. The data contained within a data lake is in its real natural state, not accurate, no insights, just data. Some features of data lakes include:
- All data is loaded from source systems. No data is turned away.
- Data is stored at the leaf level in an untransformed or nearly untransformed state.
- Data is transformed, and schema is applied to fulfill the analysis needs.
- Data lakes retain all data.
Since data lakes are designed for storing massive amounts of data in its raw form, they may be of use to government agencies who ingest vast amounts of data about citizens in Australia from say, a census, then keep that on hand until specific analysis needs to be done on one of those data sets.
How to start a data lake initiative
A data lake is the answer to organising large volumes of data from diverse sources. Today more than ever, Australian businesses are facing the universal challenge of managing exponentially growing data sets. Thankfully, the data lake landscape is evolving quickly and adding real business value to a wide range of industries - from healthcare, retail and banking to mining, manufacturing, transportation and many more.
Using an advanced modern solution for data replication, allows organisations to handle all the connections from multiple source systems to your data lake, allowing you to achieve your goals and enable increased efficiency and accuracy without a negative financial impact.
Typically, to start with a data lake project, you have to move your current data from your system through a full load process, or the "Refresh Process," allowing you to schedule how and when that data loads. For instance, you can schedule to automatically data load in batches overnight, so it's fresh and up to date at the start of business every day.
This thoughtful loading process can help prevent possible network or user issues, making your data lake creation as smooth and painless as possible.
With all your current data stored in your new data lake system, you can now take advantage of the functionalities such as Change Data Capture (CDC), capturing only the changed data using log-reading technology.
There's no risk in learning more about how this ground-breaking solution can help you use the massive amount of data at your fingertips to exact substantial, strategic improvements to your business.