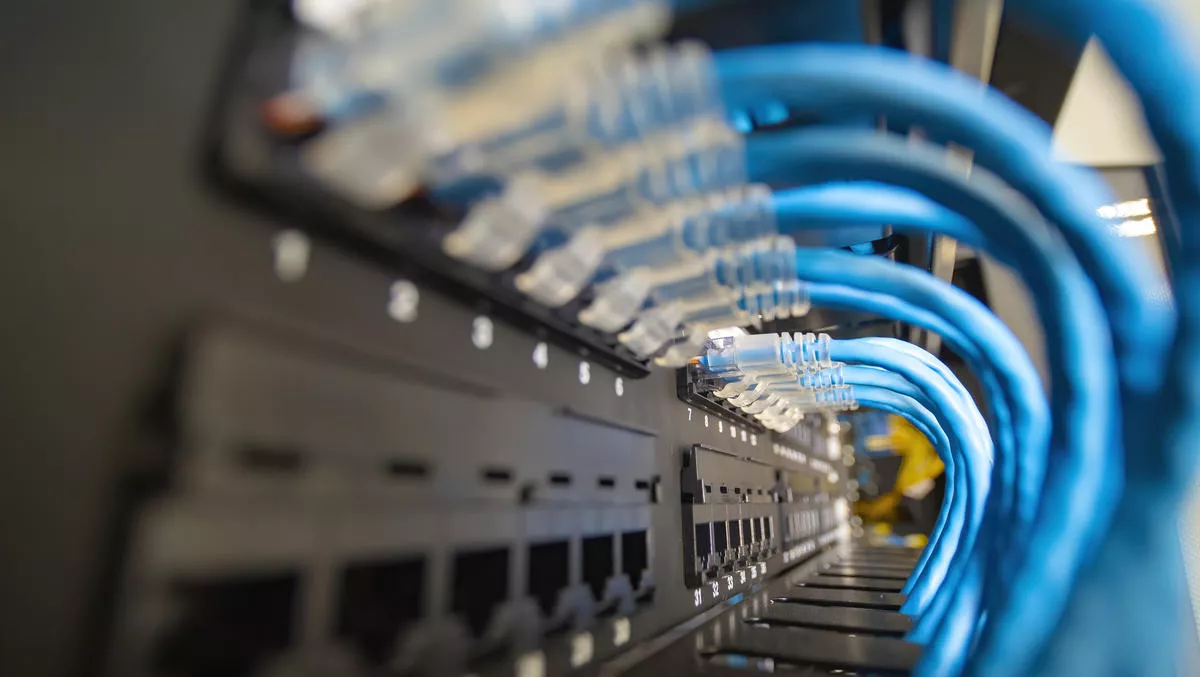
How memory failure prediction keeps data centers and the digital economy up and running
It was approximately four years ago when I wrote in an industry publication, "Today's data centers are the modern equivalent of railroad infrastructure and the world's business rides upon its rails."
Looking back, one can't help but think how understated, if not quaint, the idea seems now.
Just consider the historic surge in digital services we've witnessed as global populations were forced to work, study, socialize, conduct retail transactions, entertain themselves and even meet with healthcare providers, all from home. As Microsoft CEO Satya Nadella famously said roughly sixty days into the global health crisis, "We've seen two years' worth of digital transformation in two months."
Lest we forget, all that streaming and social media, video conferencing, cloud collaboration platforms, eCommerce, telehealth and online gaming rely on highly-available data centers as well as reliable server hardware. Forget railroad tracks. The data center, now rightly classified by governments worldwide as essential critical infrastructure, has become for business and society what oxygen is for the ultramarathon runner.
The critical difference is that we're presently in a race where no clear finish line has yet to emerge, as company after company have announced they won't be reopening their offices until mid-2021, at the earliest. Some lockdowns have returned, and much of our collective professional and personal lives remain virtual. More than ever, our data centers, and the hardware that resides there, need to stay online so that the digital economy stays up and running.
The breath of business continuity
According to the Uptime Institute's 2020 data center survey, "outages are occurring with disturbing frequency, and bigger outages are becoming more damaging and expensive" than in previous years.
In 2020, a greater percentage of outages cost more than $1 million (now, nearly one in six, compared to one in 10, as in 2019), and a greater percentage cost between $100,000 and $1 million (28% in 2019 vs 40% in 2020).
As one of the top-three hardware failures that occur in data centers, memory failures have a direct impact on server reliability. Moreover, a memory failure can have a devastating effect without giving data center operators an early enough warning of a future outage in order to take preemptive action.
Using machine learning to analyze real-time memory health data makes it possible to predict such failures ahead of time. A method of data analysis that automates analytical model building, machine learning uses algorithms that iteratively learn from data, thus allowing computers to find hidden insights without being explicitly programmed on where to look for them.
The ability to analyze real-time memory health data and avert memory failures ultimately translates to a better experience for customers. This is especially so for organizations such as online services platforms and cloud service providers, which rely heavily on server hardware reliability, availability and serviceability. These are the very types of businesses that are experiencing soaring demand today.
By deploying a memory failure prediction solution in their data center and integrating it into their existing management systems, IT staff can analyze their server memory failures, reduce downtime, and improve their current Dual Inline Memory Module (DIMM) replacement policies.
Such a memory failure prediction solution uses machine learning to analyze server memory errors down to the DIMM, bank, column, row, and cell levels to generate memory health scores for each DIMM. Changes in the health score over time can signal issues well before impact, giving enough lead-time to move a workload and or take other actions.
To get a better picture of just how the memory health score is generated, it's essential to understand that the memory failure prediction engine is placed in firmware and receives alerts when memory errors occur. When servers have a burst of errors in a specific memory region, the DIMM Health Assessment Model (DHAM) is checked to assess if the affected DIMM's health score needs to be modified. If so, then the score is changed accordingly and passed on to the baseboard management controller (BMC). This monitoring technique has been extremely useful, resulting in strong ROI, as several case studies have documented.
Memory failure prediction in action
In one case study, 'Intel Memory Failure Prediction Improves Reliability at Meituan', a Beijing company, whose online platform connects consumers with local businesses, monitored the health of the memory modules of its servers by integrating the memory failure prediction solution into their existing data center management solution.
The initial test deployment indicated that if the company deployed the solution across its full server network, server crashes caused by hardware failures could be reduced by up to 40%, which ultimately would deliver a better experience for hundreds of millions of its customers and local vendors.
In another Intel case study, 'Intel Memory Failure Prediction at Tencent', a leading China-based cloud solutions provider test deployed the memory failure prediction solution across thousands of its servers to reduce downtime caused by server memory failures. The memory failure prediction solution deployment resulted in improved memory reliability due to predictions based on the capture of micro-level memory failure information from the operating system's error detection and correction (EDAC) driver, which stores historical memory error logs.
The memory failure prediction solution also gave the cloud service provider's IT staff enough information to proactively address potential memory issues, and replace failing DIMMs before they reach a terminal stage and cause server failures, thus reducing downtime.
The cloud provider's test deployment of the memory failure prediction solution indicated a five-fold improvement on DIMM level failure prediction. If the company were to deploy the memory failure prediction solution across its entire data center portfolio, they would improve the effectiveness of server reliability aware workload management and decrease the percentage of uncorrectable errors (UEs), thereby significantly reducing downtime.
Online retailing and cloud technologies have significantly disrupted the retail and consumer goods vertical, leading to increased adoption of cloud computing. Moreover, as world events drive companies to accelerate their digital transformation initiatives practically overnight, ResearchandMarkets projects the global cloud computing market size will increase at a compound annual growth rate (CAGR) of 17.5%, surging from $371.4 billion in 2020 to $832.1 billion by 2025.
As cloud providers and retailers, along with financial services, IT, telecom, media firms and more navigate the 'next normal', maintaining data center uptime — the very breath of business continuity — has never been so business-critical.