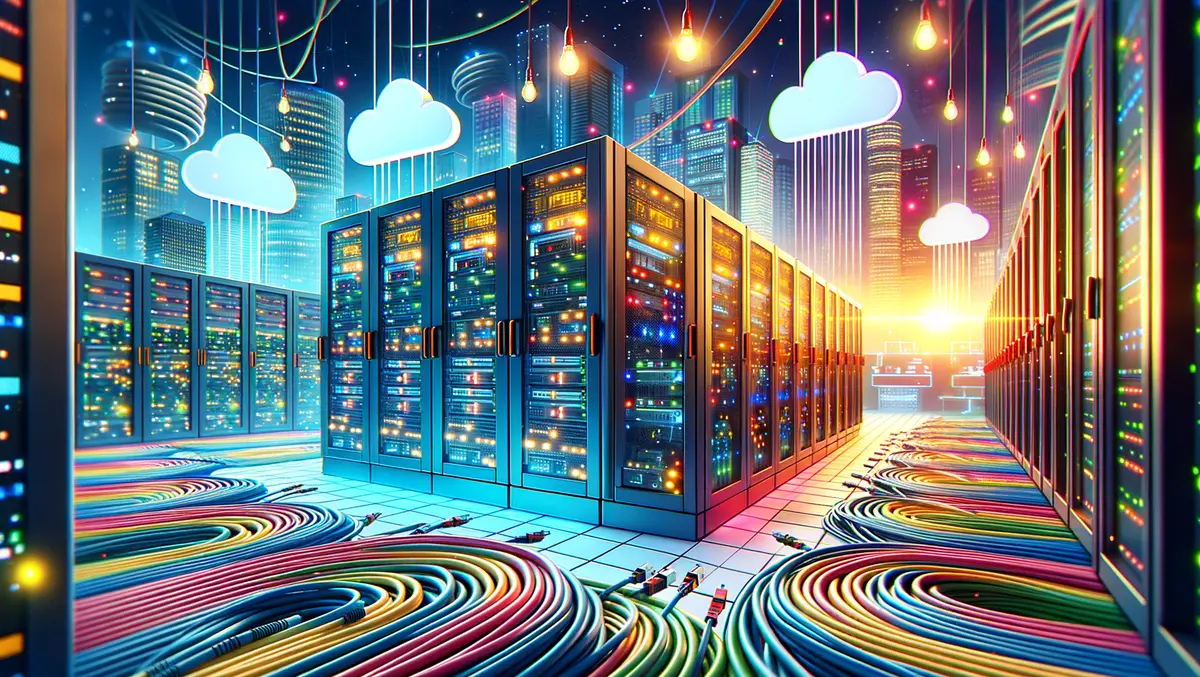
CommScope highlights key cabling considerations for AI data centers
In recent years, the landscape of artificial intelligence (AI) has dramatically evolved, pushing the boundaries of what technology can achieve and transforming the infrastructure required to support it. A key aspect of this transformation is the architecture of AI data centers, which must adapt to the unique demands of AI computation. This article delves into CommScope's cabling considerations for AI data centers, exploring the challenges and best practices essential for optimizing performance and efficiency.
The Shift Towards AI-Driven Data Centers
The proliferation of AI technologies, exemplified by innovations like DALL-E 2 and ChatGPT, has significantly impacted the public's perception and expectations of AI. As these technologies become more integral to various sectors, the infrastructure supporting them must also evolve. AI is now a primary driver for the growth of data centers, necessitating a shift in how these centers are designed and operated.
AI computation relies heavily on graphical processing units (GPUs), which are specialized for parallel processing. The sheer processing capacity required to train and run AI models often exceeds the capabilities of a single machine, necessitating multiple interconnected GPUs across servers and racks. This setup forms AI clusters within data centers, presenting distinct cabling challenges and opportunities.
Architectural Differences: AI vs. Traditional Data Centers
Traditional data centers, especially hyperscale facilities, typically employ a folded Clos architecture, also known as a "leaf-and-spine" architecture. In this setup, server racks connect to a top-of-rack (ToR) switch, which then connects to leaf switches via fiber cables. However, AI clusters demand a different approach due to their higher connectivity requirements between servers and the significant power and heat generated by GPU servers.
As outlined in the report, "GPU servers require much more connectivity between servers, but, due to power and heat restraints, there are often fewer servers per rack. The result is more inter-rack cabling in an AI data center architecture than in a traditional architecture." This increased cabling complexity is necessary to support the higher data transfer rates required by AI workloads, which range from 100G to 400G over distances that copper cables cannot support.
Practical Examples: NVIDIA's AI Data Center Architecture
A prime example of AI data center architecture is provided by NVIDIA, a leader in the AI hardware space. Their latest GPU server, the DGX H100, features multiple high-speed fiber ports for connectivity. A single DGX SuperPOD, a cluster containing 32 GPU servers, requires 384x400G fiber links for switch fabric and storage, along with 64 copper links for management. This setup illustrates the substantial increase in fiber links compared to traditional data center architectures.
Minimizing Latency in AI Clusters
Latency is a critical factor in AI and machine learning (ML) algorithms, with significant portions of the time required to run large training models attributed to network latency. As noted in the report, "One estimate claims that 30% of the time to run a large training model is spent on network latency, and 70% is spent on compute time." To minimize latency, AI clusters aim to keep GPU servers in close proximity, with nearly all links limited to 100-metre reaches.
However, not all data centers can accommodate such configurations, especially older facilities with lower power capacities. These centers may need to space out GPU racks, further complicating the cabling requirements.
Choosing the Right Transceivers and Fiber Cables
Selecting appropriate optical transceivers and fiber cables is crucial for cost and power efficiency. The report highlights the advantages of parallel optics, which do not require the optical multiplexers and demultiplexers used in wavelength division multiplexing (WDM). For instance, 400G-DR4 transceivers with eight-fiber cables are more cost-effective than their 400G-FR4 counterparts.
Additionally, the choice between single-mode and multimode fiber is influenced by cost and power considerations. Although single-mode transceivers have become more affordable, multimode transceivers remain less expensive and consume less power. This difference can result in significant savings, especially in large AI clusters with hundreds of transceivers.
Active Optical Cables vs. Transceivers with Fiber Cables
Active Optical Cables (AOCs) are commonly used in AI, ML, and high-performance computing (HPC) clusters. These cables integrate optical transmitters and receivers, offering an all-in-one solution. However, AOCs lack the flexibility of separate transceivers and fiber cables, making them less suitable for future upgrades and more prone to failure.
The report concludes that "carefully considering the AI cluster cabling will help save cost, power and installation time, enabling organizations to fully benefit from AI." By addressing the unique cabling needs of AI data centers, operators can ensure their facilities are equipped to handle the demands of current and future AI workloads.
As AI continues to drive data center growth, the architecture and cabling of these facilities must evolve to meet new challenges. By adopting best practices and optimizing cabling infrastructure, data centers can enhance performance, reduce costs, and support the next generation of AI innovations.